Abstract
The recent Ghibli art trend is an example of AI art - synthetic images or media created using Artificial Intelligence models. What might initially seem harmless, in reality, affects the political, social, and economic fabric of society in many ways. Cases involving deepfakes have grown exponentially and a social movement has started against synthetic media. Such issues require immediate action which primarily involves separating artificial media from real media.
Introduction
Images created or modified using AI models are known as synthetic images. Recent developments in Artificial intelligence have made such images more "real." This leads to confusion and chaos between what is real and what isn't, calling for an effective technique or method to label artificial images and separate them from real images.
This paper aims to take a closer look at the following topics.
Effects of AI Images on Democracy, Art, and Social Perception.
Understanding the importance of labeling AI content.
Use of labels, like disclaimers, descriptions, and bylines.
Uses and limitations of AI detectors.
The C2PA initiative and its role in separating AI-generated content.
Important concerns with the C2PA initiative.
SWOT analysis of the several mentioned solutions.
AI Images
All artificial images are created by AI software from pre-existing data sets. This means that an AI model can't create an image from scratch. It needs to be trained by thousands of real images for it to create similar images. Even deep fakes use AI to create audio or video resembling reality. All of them are trained by real pictures. This becomes important in understanding some harmful effects of AI media.
Effects of AI images
Politics and Democracy
Synthetic media has been recognised for their extensive use in politics. According to a recent report from McAfee, more than 75% of Indians encountered deepfake or synthetic content during the election campaign of 2024.
This problem is also common in other countries. Many politicians were accused of using synthetic content to further their own election campaigns or to maliciously frame other politicians. Some have even used "deep fake" and "synthetic content" as an excuse for any real scenarios where they might have been caught using offensive language. With little to no labeling of fake content, it has become nearly impossible to separate the real from the artificial. This becomes a matter of concern for democracies as a whole.
Art
AI art has become an important topic of discussion. The recent Ghibli art trend is an example. Many people have used Open AI's GPT-4o Model to convert real images into animated pictures resembling the famous art style of "Studio Ghibli". This new trend is encouraged by many but many artists have expressed concern over such art. Even the co-founder of "Studio Ghibli", Hayao Miyazaki called AI art, "an insult to life".
Historically, on a larger scale, artists always opposed the use of AI art. They have launched movements to boycott such art and to preserve the rights of artists. What seems as a matter of opinion is in reality a matter of legality. Most AI companies train their models using copyrighted art, without consent from creators of such art, and without compensating them. The Mozilla Foundation looked at 47 Large Language Models released between the years 2019 and 2023, and found that 64 percent of them were trained on a data set called common crawl which included copyrighted works. Artists are concerned that such AI models not only steal their art but affect their profession as well.
The low cost and ease at which AI models generate art directly compete with artists whose copyrighted works are being used to train the models. Furthermore, such models motivate artists to keep away from posting their art online in fear of it being used by AI models without their consent, therefore directly affecting their livelihoods and sources of income. According to the Yale Daily News, a study involving 500 Yale University Students showed that only 54 percent of them could tell if an art piece was real or synthetic. This shows that AI art is getting more realistic and will eventually affect the profession, an issue that needs immediate solutions like watermarking, or labeling to protect and preserve the rights of artists.
The attribution fallacy
In the context of AI models, the attribution fallacy is when people overemphasize the abilities of AI models while ignoring the role or capabilities of real human intelligence. Like other technological advancements, the hype around the development of AI models has created wrong perspectives on the abilities of such models. Most people, believing AI content is true, have relied on it in many cases however, there have been many issues where AI content was either inaccurate or biased. For example, in the Gender Shades project, AI-based commercial gender classification systems were tested and significant disparities in accuracy were found across different genders and skin types. Certain facial recognition tools have also been found to fail on the faces of darker-skinned females.
Unlike the popular belief, AI models cannot actually “create” images or text on their own. The use of Anthropomorphic or human terms like “Understands”, or “reasons”, with AI models overstates their abilities and leads people to believe that AI models are reliable and “Human”, what has now been termed as the “Wishful Mnemonics Fallacy”. As mentioned before, AI models are trained using data sets containing thousands of images, to enable them to recreate similar images. Therefore, in reality, AI models cannot create images from scratch. Unlike humans, it does not possess the ability to think creatively.
This misconception about AI models having an accuracy of 100 percent is harmful to real content. Many content creators reported that their content, which includes real videos or audio, is being accused as Artificial, primarily due to increased exposure to AI content. Therefore, it becomes necessary to understand the true abilities of Artificial Intelligence and to label AI content, to ensure that people are not wrongly accused of faking images or media.
Possible solutions
These issues require immediate and effective solutions. Most solutions are built on the idea of labeling AI content, that is, to effectively separate synthetic and real content. Many of these solutions, as well as their limitations, have been analysed below.
Labeling AI content
According to an AI test by Tidio, only 30 percent of the people were able to recognise a fake human portrait, and 49 percent identified music from Bach as "AI-generated music". With AI models getting better at generating realistic content, it will become extremely hard to differentiate between real and artificial content. This calls for an effective method of labeling AI content.
Disclaimers:
Descriptions
Often, some creators choose to warn their viewers that their content is generated by AI through the titles and descriptions of their videos or photos on social media platforms. Such descriptions are built around a sense of trust with the creator of the content and allow people to differentiate between real and artificial content.
Bylines:
Bylines, which usually apply to magazines or news organisations are often used in articles to credit the role of AI in an article or a post.
Pre-Roll clips:
Pre-roll clips are sometimes added to videos especially on social media platforms like YouTube to credit the role of AI in making the videos.
Side by Side comparison:
A side-by-side comparison of real and synthetic content could also be used to create an instinctive feel to help people differentiate between real and synthetic content.
Filters or tags:
Many social media platforms also mandate people to disclose whether their content involves AI-generated content. This is usually optional. The content is then accordingly labeled as "altered", "synthetic", or "AI-Generated".
Limitations:
Removal of labels:
AI labels do not travel with the content or images. Disclaimers, tags, or descriptions are removed by downloading the content, and pre-roll clips could just be edited out. This reduces their reliability as an effective method of labeling AI content.
A lack of disclosure from content creators:
Most content creators do not disclose whether their content involves AI-generated content for the following reasons:
They believe that it is obvious that their content is AI-generated
They fear it could reduce the credibility of the content.
They could be using their content or posts for malicious purposes.
Irrespective of the reasons, it is important that creators are motivated or mandated to affirm any role of AI in the generation of their content. Even though certain platforms like YouTube do this, most creators don't and get away with it.
The terms used as Disclaimers:
The effectiveness of a disclaimer depends on the specific term used to label the AI-generated content. The context, use, and efficacy of the term depend on several factors like age, geographical location, and more importantly, the general perspective on such content. MIT researchers surveyed more than 5100 people across five countries in four languages. The people were asked to find which term they believed aligned with certain content. Nine terms, often associated with AI-Generated content were used for the study- "AI generated", "generated with an AI tool", "synthetic", "manipulated", "deepfake", "edited", "not real", "AI manipulated", and "artificial".
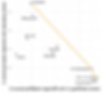
The above graph summarizes the results of the study.
It shows that people closely associated terms like "AI generated" with content generated using AI models, and terms like "deepfake" with misleading content. Therefore, while labels like AI-generated would be most effective at the primary objective, that is, labeling AI content like AI images, it has been found to be less effective in determining whether a person would share an AI-generated image. This shows a lack of awareness about how AI-generated content could be factually wrong or misleading. Social perception, therefore, is an important challenge to overcome in labeling AI content.
Geographical locations also affect the efficacy of a term. Researchers of the MIT study found that contrary to languages like English, Spanish, or Portuguese, Chinese speakers associated the word "Artificial" with human involvement. Therefore, it is important to account for multiple cultures and languages and collect data on a global scale to identify the best terms to use as disclaimers.
SWOT Analysis of the effectiveness of labels
Strengths
| Weaknesses
|
Opportunities
| Threats
|
AI detectors:
AI detectors are tools used to identify content generated using AI models to help differentiate AI-generated and real content.. Several social media platforms employ AI detectors to detect if a piece of content was generated by an AI model.
AI detectors of platforms like Youtube or TikTok work by extracting demographic or personal data from images. This includes Age, gender, time of the day and other elements like food. Such detectors use Machine Learning Algorithms and are trained over thousands of images generated by AI image generators like Dall-E, Stable diffusion, and many more.
In 2024, Youtube also announced that they are working on a technology to help people detect AI-generated content showing their faces, that is, deepfakes, to prevent the spread of misinformation.
Limitations:
Poor accuracy
Most AI detectors have very low accuracy rates which also vary depending on the type of content. Some detectors work well in determining whether an image was generated by an AI model and others could work better at determining whether an image was taken by a human. This could lead to varying answers among multiple AI models. Many content creators and artists, withhold from posting their art, or images in fear of it being flagged as AI- Generated.
To understand how well detectors work at determining whether a piece of content was AI-generated, I used five sets of images, each set consisting of an AI-generated and a similar real image. Each set was a particular type of image. The first set included images of a landscape, the second, a food item, the third, an animal, the fourth, people, and the fifth, a public space like an office.
These five sets of images were tested with five popular AI detectors. The following Bar graph summarises the results of the test.

Although the test was carried out with a small data set, the inaccuracy and inconsistency, particularly in the detection of AI-generated images, are alarming. However, most detectors were able to correctly determine the nature of a real image.
SWOT analysis of the effectiveness of AI detectors
Strengths
| Weaknesses
|
Opportunities
| Threats
|
Coalition for Content Provenance and Authenticity (C2PA)
The Coalition for Content Provenance and Authenticity, popularly known as C2PA is an initiative being led by several tech organizations like Adobe, Microsoft, and Open AI. The initiative works towards using provenance data to separate real content from AI content.
Metadata and Provenance information
Metadata is information or a description that is manually added to an image or a video. For example, when we take a photo, the location and the time are considered the metadata for that photo.
Provenance information describes the origin of a piece of content as well as the changes in the content over time. Both metadata and provenance information, could play an important role in watermarking AI content and authenticating real content.
How C2PA works
C2PA binds the image or video, its metadata like its provenance information, and a digital signature by the publisher, as assertions and credentials in a cryptographic seal called a manifest. If the image or video is changed, this change is recorded in the manifest helping us verify whether an image is real or not.

The viewer can use a manifest consumer also known as a C2PA validator to validate the credentials, assertions, and the digital signature of the image. Companies like truepic help in signing and verifying the piece of content.
Limitations
Cooperation from Companies
For proper implementation of C2PA, greater cooperation is required from organizations and companies. This has recently grown with a greater buzz over AI images. Even the chair of C2PA, Andrew Jenks announced that membership has increased by 56 percent in recent months. However, many social media platforms have not yet implemented C2PA. A lack of legal measures for implementing C2PA also contribute to their ineffectiveness at curbing AI generated content.
Anonymity
The main disadvantage with the C2PA initiative is a lack of anonymity. The C2PA initiative makes it nearly impossible for someone to post a piece of content without leaving a digital footprint. According to the C2PA website, the C2PA content credential references added to an image could also be used to track the viewers of the content against their will, contributing to an increase in malicious activities.
Such a lack of anonymity has also led to concerns over the use of C2PA for mass surveillance which includes tracking individuals as well as their personal information through metadata and provenance information.
However, C2PA does mention features to ensure protection of personal information. These features include the option to redact assertions that may contain sensitive information, as well as the opportunity to use anonymous or pseudo-anonymous identities for signing claims.
Inaccessibility:
Although C2PA intends to focus on global accessibility, it faces issues like language and financial barriers. The language versioning for C2PA enabled tools is still limited and is therefore inaccessible in many parts of the world, especially in countries like Myanmar where a typeface other than UNICODE is used. Moreover, the costs associated with buying and using C2PA-enabled tools discourage people from using the initiative.
Legal concerns
Legal concerns over the C2PA initiative include fear of enforcement of restrictive laws that use available provenance information to track content like images, eventually leading to a loss of the freedom of expression. This particularly affects journalists who could face restrictions over their creativity as well.
SWOT analysis of the effectiveness of the C2PA initiative
Strengths
| Weaknesses
|
Opportunities
| Threats
|
Conclusion
While AI can be helpful, it is necessary to understand the importance of labeling AI content, especially images, to curtail the spread of misinformation, and to help people decide what is real and what isn’t. Although there are multiple tools to label AI content, there are still significant limitations in many of them. Greater cooperation between companies and individuals is important to develop an effective mechanism to separate AI images without compromising the rights of individuals.
Bibliography
1. Buolamwini, J., & Gebru, T. (2018) Buolamwini, J., & Gebru, T. (2018). Gender shades: Intersectional accuracy disparities in commercial gender classification. In Proceedings of the 1st Conference on Fairness, Accountability and Transparency (Vol. 81, pp. 1–15). PMLR. https://proceedings.mlr.press/v81/buolamwini18a/buolamwini18a.pdf
2. Coalition for Content Provenance and Authenticity. (2021) Coalition for Content Provenance and Authenticity. (2021). C2PA Harms Modelling (Version 1.0). https://c2pa.org/specifications/specifications/1.0/security/Harms_Modelling.html
3. Coalition for Content Provenance and Authenticity. (2021) Coalition for Content Provenance and Authenticity. (2021). C2PA Security Considerations (Version 1.0). https://c2pa.org/specifications/specifications/1.0/security/Security_Considerations.html
4. Coalition for Content Provenance and Authenticity. (2021) Coalition for Content Provenance and Authenticity. (2021). C2PA Technical Specification (Version 1.0). https://c2pa.org/specifications/specifications/1.0/specs/C2PA_Specification.html
5. Epstein, Z., Fang, M. C., Arechar, A. A., & Rand, D. G. (2023) Epstein, Z., Fang, M. C., Arechar, A. A., & Rand, D. G. (2023, July 28). What label should be applied to content produced by generative AI? PsyArXiv. https://doi.org/10.31234/osf.io/v4mfz
6. Interaction Design Foundation. (2025) Interaction Design Foundation. (2025). AI-generated art. https://www.interaction-design.org/literature/topics/ai-generated-art
7. Karamotchev, P. (2024) Karamotchev, P. (2024, August 2). The overestimation of AI technology. INDUSTRIA. https://medium.com/industria-tech/the-overestimation-of-ai-technology-c5fa1a6f728a
8. Leibowicz, C. (2023) Leibowicz, C. (2023, August 9). Why watermarking AI-generated content won’t guarantee trust online. MIT Technology Review. https://www.technologyreview.com/2023/08/09/1077516/watermarking-ai-trust-online/